Everything You Need to Know About Machine Learning
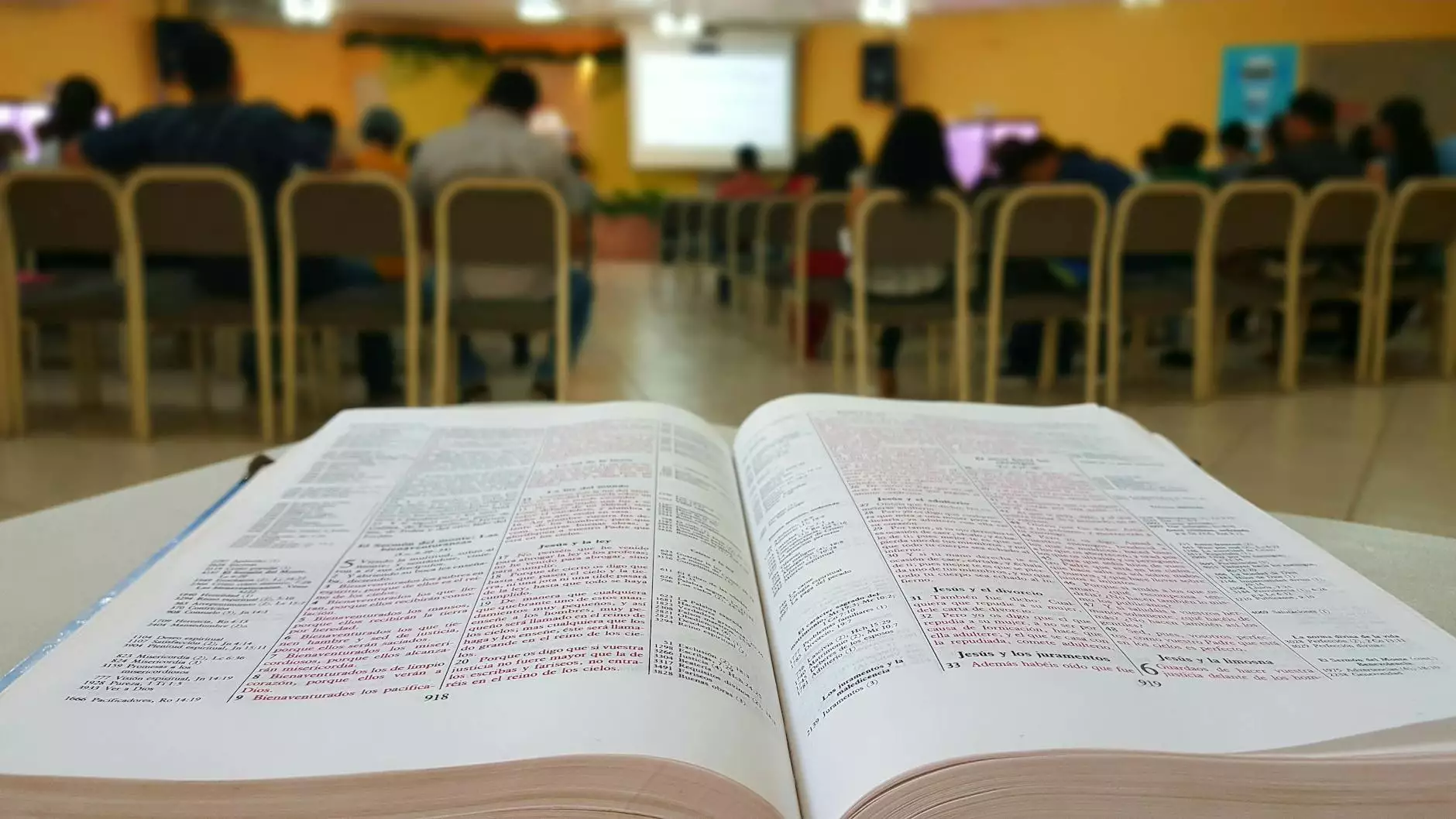
In today's rapidly advancing technological landscape, machine learning stands as a revolutionary force that is reshaping industries, enhancing decision-making processes, and creating efficiencies previously thought impossible. This article delves deep into everything you need to know about machine learning, providing an exhaustive exploration of its concepts, applications, and implications in the business world.
What is Machine Learning?
Machine learning is a subset of artificial intelligence (AI) that enables systems to learn from data, improve their performance on tasks over time, and make predictions or decisions without being explicitly programmed. Through algorithms and statistical models, machines can process vast amounts of data to identify patterns, which can be utilized in various applications.
Types of Machine Learning
Machine learning can be categorized into three main types:
- Supervised Learning: In this method, the model is trained on a labeled dataset, meaning that it learns from both the input data and the corresponding output labels. It is commonly used for classification and regression tasks.
- Unsupervised Learning: This approach involves training a model using data that has no labels. The system attempts to learn the underlying structure or distribution in the data. It is often used for clustering and association problems.
- Reinforcement Learning: In reinforcement learning, an agent learns to make decisions by performing certain actions and receiving rewards or penalties. This trial-and-error methodology is particularly effective in environments where sequences of actions are vital for achieving a specific goal.
Why is Machine Learning Important for Businesses?
The relevance of machine learning in the commercial sector cannot be overstated. Here are several key reasons why businesses should integrate machine learning into their operations:
- Data-Driven Decision Making: Machine learning equips businesses to analyze large datasets and extract actionable insights, facilitating informed decision-making and strategy development.
- Enhanced Customer Experiences: By leveraging machine learning algorithms, companies can offer personalized recommendations, improving customer satisfaction and engagement.
- Operational Efficiency: Automating repetitive tasks with machine learning can increase productivity, reduce operational costs, and allow human resources to focus on more strategic activities.
- Risk Management: Machine learning can enhance risk assessment and fraud detection, allowing organizations to predict and mitigate potential threats.
- Innovation and Competitive Advantage: Businesses that adopt machine learning technologies can develop new products and services that give them an edge over competitors.
Applications of Machine Learning in Various Industries
Machine learning has applications across a spectrum of industries, transforming the way they operate. Here are some of the most notable examples:
1. Healthcare
In healthcare, machine learning algorithms are utilized for diagnosing diseases, predicting patient outcomes, and personalizing treatment plans. Technologies such as predictive analytics help identify potential health risks, while natural language processing (NLP) enhances patient communication and documentation processes.
2. Finance
The financial industry employs machine learning to detect fraudulent transactions, assess credit risk, and optimize investment portfolios. By analyzing transaction patterns, financial institutions can identify anomalies indicative of fraud, thereby protecting both themselves and their customers.
3. Retail
In the retail sector, machine learning enriches the shopping experience through personalized recommendations and targeted marketing strategies. Retailers analyze customer behavior to predict future purchases and adjust inventory levels accordingly, enhancing customer satisfaction and operational efficiency.
4. Manufacturing
Machine learning facilitates predictive maintenance in manufacturing, reducing downtime and extending equipment lifespan. By predicting equipment failures before they occur, companies can schedule maintenance proactively, thus saving costs and ensuring production continuity.
5. Transportation
In transportation, machine learning is the backbone of autonomous vehicles. It processes real-time data from sensors to make instantaneous decisions, ensuring safety and efficiency on the roads. Ride-sharing services leverage machine learning for route optimization and demand prediction.
Challenges in Machine Learning Implementation
While the benefits of machine learning are substantial, implementing these technologies comes with challenges:
- Data Quality: The effectiveness of machine learning algorithms heavily depends on the quality of the data used. Poor data quality can lead to inaccurate predictions and insights.
- Complexity of Algorithms: Developing and fine-tuning machine learning models can be complex and requires skilled data scientists.
- Integration with Existing Systems: Integrating machine learning solutions into existing business processes and systems presents challenges, particularly if those systems are outdated or not designed for advanced analytics.
- Ethical Considerations: The use of machine learning raises ethical concerns, especially concerning data privacy, surveillance, and algorithmic bias that organizations must address proactively.
Future Trends in Machine Learning
The field of machine learning is evolving rapidly. Here are some future trends to watch:
- Explainable AI: As businesses adopt machine learning, the demand for explainable AI is growing. Stakeholders want clarity on AI decisions to ensure transparency and trust.
- Federated Learning: This decentralized approach enables devices to collaboratively learn from data without needing to exchange sensitive information, enhancing privacy.
- Automation of Machine Learning (AutoML): Tools that automate the machine learning process are making it easier for non-experts to deploy models, democratizing access to AI technologies.
- Conversational AI: The rise of advanced chatbots and virtual assistants powered by machine learning will transform customer service across industries.
- Integration with IoT: The convergence of machine learning and the Internet of Things (IoT) will generate unprecedented insights from real-time data, driving smarter business decisions.
Getting Started with Machine Learning
For businesses looking to incorporate machine learning, consider the following steps:
- Identify Business Needs: Clearly define the business problem you wish to solve with machine learning.
- Gather and Prepare Data: Collect relevant and high-quality data, ensuring it is well-structured and clean.
- Select Appropriate Tools and Technologies: Choose the right machine learning frameworks and tools that align with your objectives and technical capabilities.
- Develop and Train Models: Build machine learning models using suitable algorithms, and train them on your dataset for optimal performance.
- Test and Validate: Rigorously test your models to validate their accuracy and effectiveness.
- Deploy and Monitor: Once validated, deploy the models into production and continuously monitor their performance over time, adapting to new data.
Conclusion
Machine learning is undeniably one of the most significant advancements in technology, offering transformative potential for businesses across various industries. By understanding everything you need to know about machine learning, organizations can harness its power to drive innovation, improve efficiencies, and gain competitive advantages. As the field continues to evolve, staying informed and adaptable will be crucial to leveraging machine learning's full capabilities in the ever-changing business landscape.
For more insights and expert guidance on integrating machine learning into your business strategy, visit machinelearningconsulting.net.